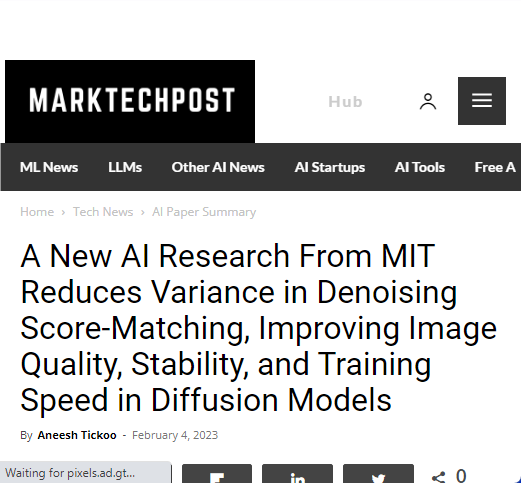
Enhancing Diffusion Model Performance: MIT’s New STF AI Research Reduces Variance in Denoising Score-Matching
A new AI research from MIT reduces variance in denoising score-matching, improving image quality, stability, and training speed in diffusion models
Recent diffusion models have produced excellent results for various generating tasks including the creations of images, molecular conformers, and 3D point clouds. Ito stochastic equations (SDEs) can be used to integrate these models. Score-matching allows the models to acquire information about time-dependent scores fields, which is then used by reverse SDEs during generative sampling. Diffusion models include the variance-preserving SDE (VP) and the variance-exploding SDE (VE). EDM is the best performance available to date. The current training method for diffusion model can be improved, despite the excellent empirical results.
The Stable Target Field objective (STF), is a variation of the Denoising Score-Matching objective. The high volatility of training targets for the denoising-score-matching (DSM) goal can lead to subpar performance. To better understand the volatility, they divided the score field in three regimes. According to the investigation, this phenomenon occurs mainly in the intermediate regime. This is defined by different modes or data points that have a similar effect on scores. In other words under this regime it is still not known where the noise samples generated throughout the forward process originate. Figure 1(a), illustrates the differences in the DSM objectives and the STF goals proposed by the STF.
Figure 1: Comparisons between the DSM objectives and our suggested STF objectives.
Source: