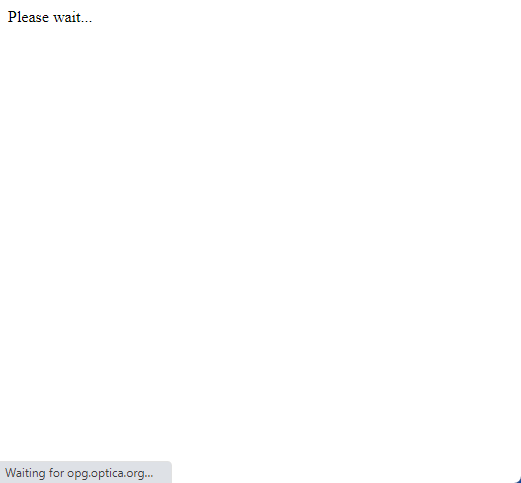
Unsupervised Layer-Based Computer-Generated Holography with a Diffraction Model-Informed Neural Network
A neural network based on a Diffraction Model for layer-based unsupervised computer-generated holography
The learning-based computer generated holography (CGH), which enables real-time holographic display, has shown great promise. A large dataset of target images and their holograms is required for supervised CGH. We propose a neural network framework (self holo) based on diffraction models for 3D phase only holograms. The neural network can be trained unsupervised without a labeled data set, as the angular propagation is incorporated in the network. The complexity of the self holo is independent of how many depth layers are used. This is achieved by randomly reconstructing holograms from different representations of 3D objects and using them to create a hologram on one layer. Self-holo uses depth and amplitude maps as input to create a 3D or 2D hologram. We show 3D reconstructions that have a good 3D appearance and demonstrate the generalizability and applicability of self-holo to numerical and optical experiments.
Source:
https://opg.optica.org/oe/fulltext.cfm?uri=oe-30-25-44814&id=521932