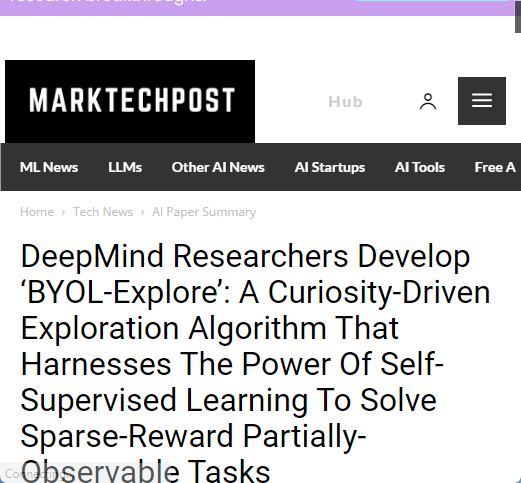
Title:Exploring Sparse-Reward Partially-Observable Tasks with BYOL-Explore: A Curiosity-Driven Exploration Algorithm Leveraging Self-Supervised Learning
DeepMind Researchers Create ‘BYOL Explore’: An Exploration Algorithm Driven By Curiosity That Harnesses Self-Supervised learning To Solve Sparse Reward, Partially Observable Tasks
DeepMind Researchers Create ‘BYOL Explore’, An Exploration Algorithm Driven By Curiosity That Harnesses Self-Supervised learning To Solve Sparse Reward Partially Observable Tasks
Exploration of the environment is necessary for reinforcement learning (RL). Exploration becomes even more important when there are few extrinsic rewards or they are difficult to get. It is impossible to explore every area in rich settings because of the vast size of the environment. The question then becomes: How can an agent determine which areas in the environment are worth investigating? Curiosity-driven exploring is one viable way to solve this problem. This involves learning a world-model, which is a model that predicts specific knowledge about the universe, and (ii), exploiting the disparities between predictions and experiences to create intrinsic rewards.
A RL agent who maximizes these intrinsic motivations steers itself towards situations where the World Model is unreliable and unsatisfactory. This creates new paths for world model. The quality of exploration policy can be influenced by world model characteristics, which then helps the world models by collecting new information. It may be important to treat learning the world-model and the exploratory policies as a single problem that must be solved, rather than two separate tasks. Researchers at Deepmind took this into consideration and developed a curiosity-driven algorithm called BYOL Explore. Its appeal stems from conceptual simplicity, generality and excellent performance.
The strategy is based upon Bootstrap Your Own (BYOL), which is a self-supervised method of latent-predictive that predicts an older version of the latent representation. BYOL Explore learns the world model using a self-supervised loss prediction and then trains the curiosity-driven policies with the same loss. This bootstrapping method has been used successfully in computer vision, graph representations learning, and RL representations learning. BYOL Explore, on the other hand, goes a step further by not only learning a flexible world-model but also exploiting its loss to motivate exploration.
Source: